Data Science Applications
Clinical data science represents the convergence of healthcare, data analytics, and technology to extract meaningful insights from vast volumes of medical data. By leveraging advanced analytics and machine learning techniques, clinical data scientists aim to enhance patient care, drive medical innovation, and revolutionize the healthcare landscape.
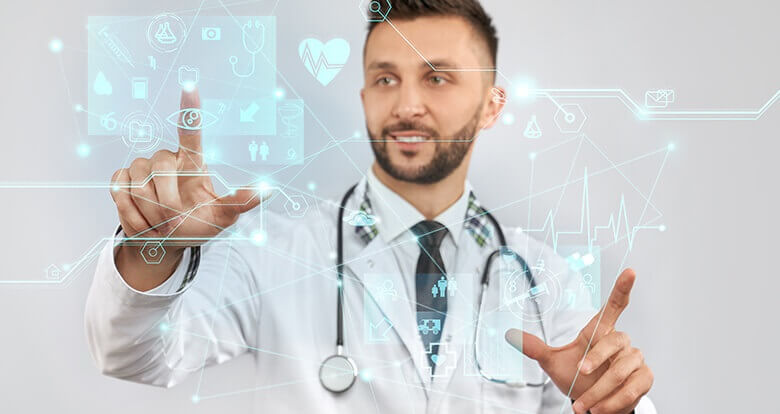
Program Highlights

Modules-
10

HRS of Learning-
300+

Weeks -
6

FAQs-
150+

Mock Interview FAQs-
100+
Program Overview
- By analyzing patient data, clinical data scientists can develop models to predict disease progression, identify individuals at higher risk for specific conditions, and customize treatment plans accordingly.
- Clinical data science plays a crucial role in pharmaceutical research. It involves mining biological data, clinical trial results, and real-world evidence to identify potential drug candidates, optimize dosages, and assess safety profiles.
- CDSS leverage clinical data to provide healthcare professionals with evidence-based recommendations for diagnosis, treatment, and patient management. These systems aid in making informed decisions at the point of care.
- Clinical data science enables the tailoring of medical treatments to individual patient characteristics, including genetics, lifestyle, and environmental factors. This approach enhances treatment efficacy and reduces adverse effects.
- By analyzing healthcare data, clinical data scientists can detect trends in disease occurrence, allowing for timely intervention and resource allocation. This is particularly critical for infectious disease control.
- Clinical data science is instrumental in extracting valuable information from medical images. It includes techniques such as radiomics, which involves the quantitative analysis of image features to inform diagnosis and treatment planning.
- NLP enables the extraction of structured information from unstructured clinical notes, medical literature, and other text-based sources. This technology supports clinical research, decision-making, and automated coding.
- Clinical data science plays a vital role in analyzing data from wearable devices and remote monitoring systems. This allows for continuous monitoring of patient health and early detection of potential issues.
- Clinical data scientists work with genomic data to identify genetic variations associated with disease susceptibility, drug response, and treatment outcomes. This information is crucial for precision medicine initiatives.
- Data-driven approaches help healthcare providers optimize resource allocation, streamline workflows, and improve operational efficiency.
The field of clinical data science is dynamic and continually evolving. As technology advances and healthcare data becomes more accessible, clinical data scientists are poised to play an even more prominent role in shaping the future of healthcare.
However, challenges such as data privacy, interoperability, and the need for robust regulatory frameworks remain areas of focus for professionals in this field. In summary, clinical data science applications represent a powerful force in advancing healthcare. By harnessing the potential of data, clinical data scientists are driving innovation, improving patient outcomes, and revolutionizing the practice of medicine.
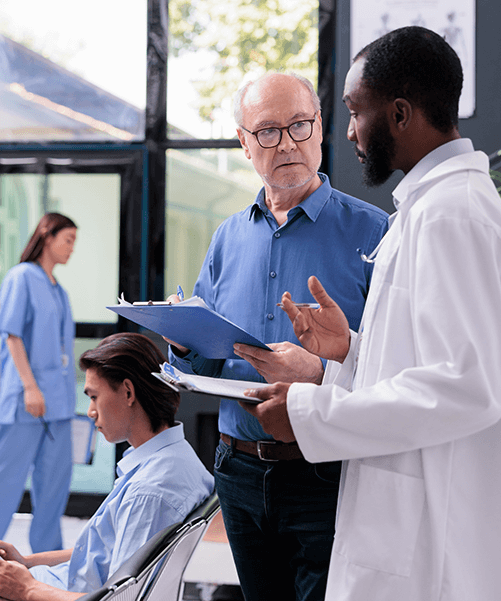
Program Objectives
The objectives for a Clinical Data Science Applications program are designed to equip participants with the knowledge and skills necessary to effectively apply data science techniques in the healthcare domain. Here are the key objectives for such a program:
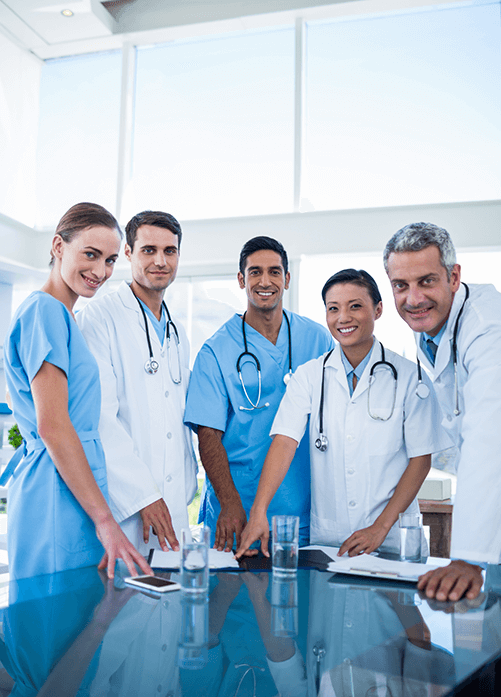
- Gain a comprehensive understanding of the healthcare industry, including its structure, stakeholders, and challenges.
- Learn to work with various sources of clinical data, such as Electronic Health Records (EHR), medical imaging, wearable devices, and genomic data.
- Acquire skills to clean, transform, and prepare healthcare data for analysis, addressing issues like missing values, outliers, and data quality.
- Use statistical methods to explore and analyze healthcare data, including descriptive statistics, hypothesis testing, and survival analysis.
- Develop proficiency in using machine learning algorithms to build predictive models, classify diseases, and optimize treatment plans.
- Apply NLP techniques to extract information from clinical text, automate coding, and analyze unstructured healthcare data.
- Gain expertise in processing and analyzing medical images for tasks like segmentation, detection, and quantitative analysis.
- Understand how to work with genomic data, identify genetic variations, and use genomic information to personalize treatment approaches.
- Learn to develop systems that provide evidence-based recommendations to aid healthcare professionals in diagnosis and treatment decisions.
- Build models to predict patient outcomes, identify high-risk individuals, and optimize treatment strategies.
- Apply data science techniques to monitor disease trends, detect outbreaks, and support public health interventions.
- Explore how data science can enhance remote patient monitoring, improve telehealth interventions, and enable proactive healthcare delivery.
- Understand and uphold ethical considerations in healthcare data usage, ensuring compliance with regulatory frameworks (e.g., HIPAA).
- Develop the ability to communicate findings and collaborate effectively with healthcare professionals, researchers, and stakeholders.
- Keep abreast of the latest technologies, tools, and methodologies in clinical data science applications.
By achieving these objectives, participants will be well-prepared to contribute to the advancement of healthcare through the intelligent use of data science techniques and applications.
Suggested For
- Medical graduates with MBBS, MD, DO, Ph.Ds',
- Dental graduates with BDS, MDS, DMD, DDS
- Pharmacy graduates with B.Pharm, PharmD
- Ph.D
- Nursing graduates with RN, BS, LPN
- Majors in Biological Sciences/Life Sciences/allied health fields.
- Pharmaceutical Quality analysts
- Microbiology, Biochemistry, Organic Chemistry and Biotechnology students
.
- Health Informatics
- Regulatory affairs
- Medical Affairs
- Clinical Research
- Clinical Data Management or Data sciences
- Clinical Trial Management
- Quality Assurance
- Quality Control
- Pharmacovigilance and Drug Safety
Program Curriculum
Designing a comprehensive curriculum for Clinical Data Science Applications involves structuring a program that covers key theoretical concepts and provides hands-on training in practical skills. Here's a suggested outline for a Clinical Data Science Applications curriculum:
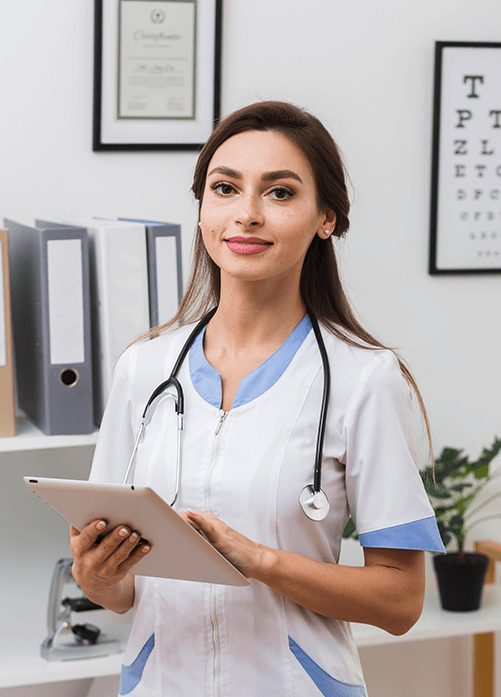
- Definition and Scope of Clinical Data Science
- Importance and Applications in Healthcare
- Regulatory Framework and Ethical Considerations
- Electronic Health Records (EHR) and Health Information Systems
- Medical Imaging Data and Analysis
- Wearable Devices and Remote Monitoring
- Data Cleaning Techniques for Healthcare Data
- Handling Missing Values and Outliers
- Data Normalization and Standardization
- Descriptive Statistics and Data Visualization
- Inferential Statistics and Hypothesis Testing
- Survival Analysis and Time-to-Event Data
- Introduction to Machine Learning Algorithms
- Predictive Modeling for Patient Outcomes
- Disease Classification and Risk Assessment
- Text Mining and Information Extraction
- Clinical Text Processing and Annotation
- Clinical Coding and Terminology Standardization
- Image Preprocessing and Feature Extraction
- Segmentation and Detection Techniques
- Radiomics and Quantitative Imaging
- Introduction to Genomic Data and Sequencing Technologies
- Variant Calling and Genome Annotation
- GWAS and Association Studies in Clinical Research
- Components and Architecture of CDSS
- Rule-Based and Machine Learning-Based CDSS
- Evaluation and Integration into Clinical Workflow
- Predictive Modeling for Disease Outbreaks
- Resource Allocation and Capacity Planning
- Early Warning Systems for Patient Deterioration
- Remote Patient Monitoring Technologies
- Data Integration and Analysis for Telehealth
- Impact of Telehealth on Healthcare Delivery
- Patient Privacy and Data Security
- Compliance with Regulatory Standards (e.g., HIPAA)
- Informed Consent and Ethical Use of Healthcare Data
- Apply Data Science Techniques to Solve a Clinical Problem
- Analyze Real-world Healthcare Data
- Present Findings and Recommendations
- Quizzes and Knowledge Checks
- Practical Exercises (e.g., Data Analysis, Machine Learning Models)
- Group Projects and Presentations
- Final Capstone Project Evaluation
- Guest Lectures from Industry Experts
- Hands-On Labs with Healthcare Data
- Mock Clinical Data Science Applications
Remember to adapt and update the curriculum to incorporate the latest technologies, regulatory guidelines, and industry best practices in Clinical Data Science Applications.
Career Paths
Clinical Data Management (CDM) is a critical component of clinical research, involving the collection, cleaning, and management of data from clinical trials. Here are some common career paths in Clinical Data Management:
- Responsibilities: Assist in data collection, validation, and cleaning activities. Ensure data quality and compliance with protocols.
- Skills Needed: Attention to detail, organizational skills, familiarity with clinical trial processes.
- Responsibilities: Oversee the data management process, develop data management plans, and coordinate data collection and validation activities.
- Skills Needed: Project management skills, knowledge of data management tools, attention to detail.
- Responsibilities: Analyze and report on clinical trial data, identify trends, and generate data summaries for reporting.
- Skills Needed: Data analysis skills, statistical knowledge, proficiency in data analysis software.
- Responsibilities: Implement and oversee data quality control processes, ensure compliance with regulatory requirements and industry standards.
- Skills Needed: Quality assurance knowledge, attention to detail, analytical skills.
- Responsibilities: Design and program electronic data capture (EDC) systems, generate data listings and reports.
- Skills Needed: Programming skills (e.g., SAS, SQL), database management knowledge, attention to detail.
- Responsibilities: Develop and maintain data standards, ensure consistency and compliance with industry standards (e.g., CDISC).
- Skills Needed: Knowledge of data standards, attention to detail, familiarity with CDISC standards.
- Responsibilities: Apply advanced data analysis techniques to generate insights from clinical trial data, contribute to study design and protocol development.
- Skills Needed: Statistical knowledge, data analysis skills, clinical research expertise.
- Responsibilities: Oversee the overall data management operations, lead a team of data managers, and ensure timely and high-quality data deliverables.
- Skills Needed: Leadership skills, project management expertise, strategic thinking.
- Responsibilities: Conduct audits to ensure compliance with data management processes, identify areas for improvement, and implement quality control measures.
- Skills Needed: Auditing skills, quality assurance knowledge, attention to detail.
- Responsibilities: Develop and implement data governance policies and procedures, ensure data integrity and compliance with regulatory requirements.
- Skills Needed: Governance knowledge, attention to detail, regulatory expertise.
- Responsibilities: Provide training on data management principles, tools, and processes to clinical research professionals.
- Skills Needed: Communication skills, knowledge of data management practices.
- Responsibilities: Offer specialized data management services to pharmaceutical companies, clinical research organizations, or research institutions.
- Skills Needed: Expertise in data management, business acumen, networking skills.
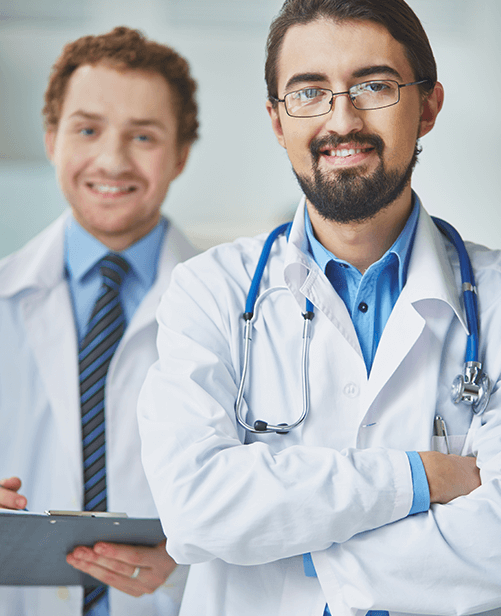
Remember that career paths in Clinical Data Management can evolve with experience and may lead to roles in project management, regulatory affairs, or other areas within the clinical research industry. Continuous professional development and staying updated with industry trends and technologies are important for success in this field.