Advanced Clinical Data Management Certificate program
Clinical Data Management (CDM) is the handling of information that results from clinical trials. All aspects of processing study information are part of clinical data management. This includes developing and maintaining software systems, databases,processes, procedures,training, and protocols to support collecting, cleaning,and managing subject or trial Data.
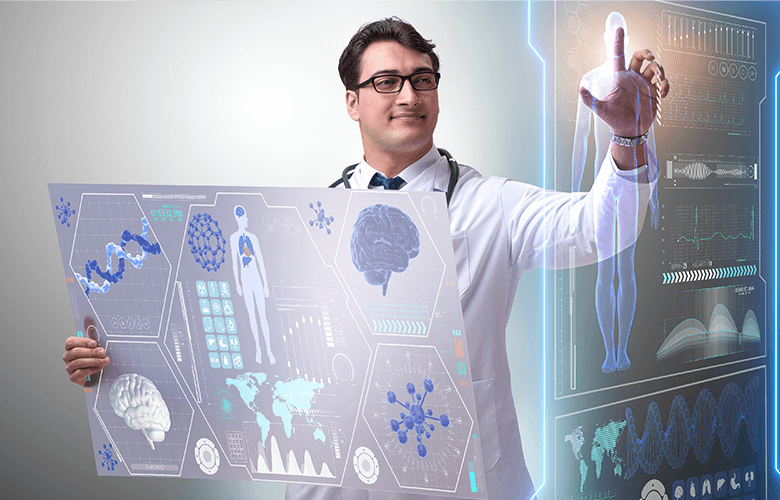
Program Highlights

Modules-
50

HRS of Learning-
500+

Weeks-
12

Interview based FAQs-
250+

Real World Scenarios - Q&A-
100+
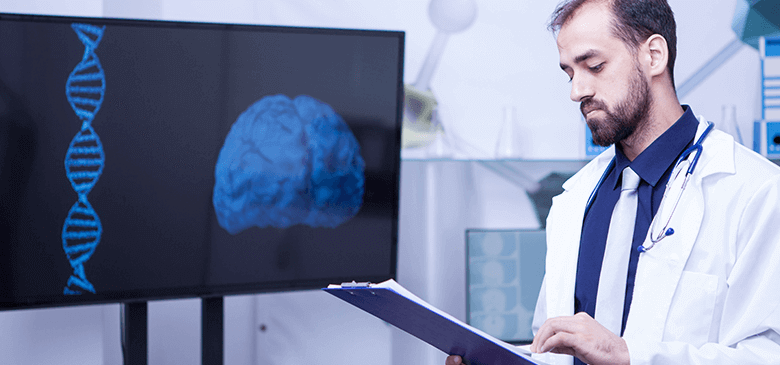
Program Overview
Clinical data science is a multidisciplinary field that applies advanced analytics, data management, and statistical techniques to extract meaningful insights from clinical data. This field plays a pivotal role in modern healthcare by transforming raw medical data into actionable knowledge, ultimately leading to improved patient care and outcomes.
Program Objectives
A Clinical Data Management program aims to equip participants with the knowledge and skills needed to effectively manage clinical trial data in compliance with regulatory standards. The program's objectives may include:
- Ensure participants grasp the fundamental concepts, processes, and best practices related to clinical data management.
- Familiarize participants with international and local regulatory requirements governing clinical data management, such as ICH E6(R2) and other relevant guidelines.
- Provide hands-on training in the use of Electronic Data Capture (EDC) systems, data validation software, and other data management tools.
- Instill the importance of maintaining data accuracy, consistency, and integrity throughout the data management lifecycle.
- Equip participants with the skills to design, develop, and manage clinical databases, ensuring they meet study requirements and regulatory standards.
- Train participants in data entry best practices, and teach techniques for identifying and resolving data discrepancies and errors.
- Emphasize adherence to GCP principles and practices in all aspects of clinical data management.
- Provide techniques for conducting thorough data validation, quality checks, and review processes to maintain data accuracy.
- Cover the collection, processing, and reporting of adverse events and safety data, ensuring compliance with pharmacovigilance requirements.
- Teach participants how to prepare for, respond to, and manage regulatory audits and inspections related to clinical data management.
- Develop skills in communication, collaboration, and coordination with cross-functional teams involved in clinical trials.
- Keep participants updated with the latest advancements in data management technologies, such as AI, machine learning, and blockchain, and their applications in clinical research.
- Emphasize ethical considerations in data management, including privacy, confidentiality, and informed consent.
- Cultivate the ability to analyze complex data management challenges and develop effective solutions.
- Offer opportunities for participants to apply their knowledge and skills through hands-on exercises, case studies, and simulations.
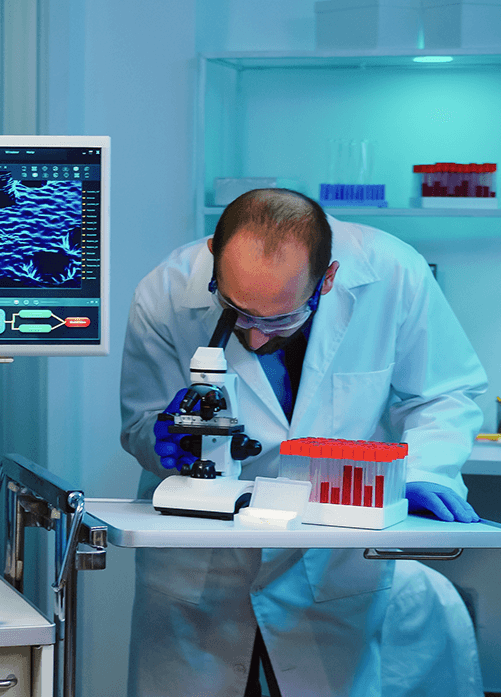
By achieving these objectives, participants will be well-prepared to take on roles in clinical data management, ensuring the integrity and accuracy of data in clinical trials, ultimately contributing to the advancement of medical knowledge and patient care.
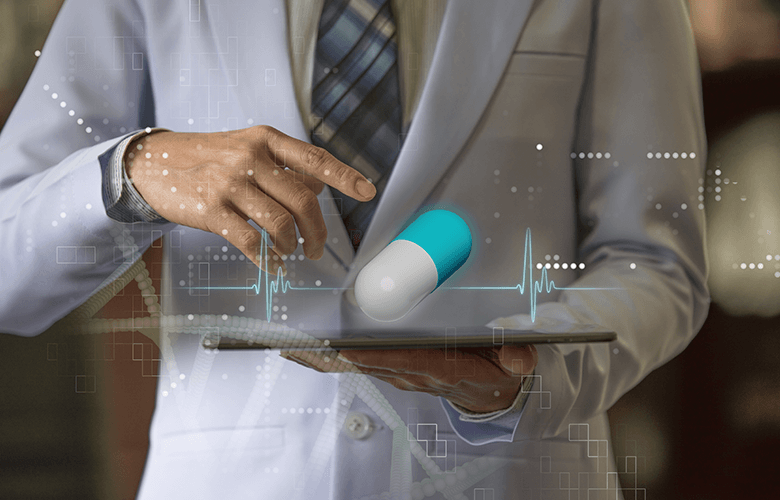
Key Components of Clinical Data Science
- Clinical data scientists are responsible for collecting, organizing, and managing large volumes of patient data. This includes electronic health records, medical imaging, laboratory results, and other sources.
- Raw clinical data often contains errors, inconsistencies, and missing values. Clinical data scientists employ techniques to clean, validate, and preprocess data, ensuring its accuracy and reliability.
- Advanced statistical methods are applied to analyze clinical data. This may involve inferential statistics, machine learning algorithms, and predictive modeling to identify patterns, trends, and correlations.
- Clinical data scientists play a crucial role in designing clinical trials. They develop protocols, select appropriate endpoints, and determine the sample size required for statistical significance.
- By utilizing clinical data, scientists can develop predictive models to assess patient risk for various conditions. These models assist in early intervention and personalized treatment planning.
- Clinical data science is integral to the pharmaceutical industry. It involves analyzing data from preclinical studies, clinical trials, and post-marketing surveillance to assess drug safety and efficacy.
- Clinical data scientists leverage real-world data from diverse sources to generate evidence outside of controlled clinical trials. This provides valuable insights into treatment effectiveness and safety in real-world settings.
- Clinical data science plays a vital role in the advancement of precision medicine. It involves tailoring medical treatments to individual patient characteristics, including genetics, lifestyle, and environment.
- These systems utilize clinical data to provide healthcare professionals with evidence-based recommendations for diagnosis, treatment, and patient management.
Target Audience
- Medical graduates with MBBS, MD, DO, Ph.Ds',
- Dental graduates with BDS, MDS, DMD, DDS
- Pharmacy graduates with B.Pharm, PharmD
- Ph.D
- Nursing graduates with RN, BS, LPN
- Majors in Biological Sciences/Life Sciences/allied health fields.
- Pharmaceutical Quality analysts
- Microbiology, Biochemistry, Organic Chemistry and Biotechnology students
.
- Health Informatics
- Regulatory affairs
- Medical Affairs
- Clinical Research
- Clinical Data Management or Data sciences
- Clinical Trial Management
- Quality Assurance
- Quality Control
- Pharmacovigilance and Drug Safety
Program Curriculum
Designing a comprehensive curriculum for Clinical Data Management involves structuring a program that covers key theoretical concepts and provides hands-on training in practical skills. Here's a suggested outline for a Clinical Data Management curriculum:
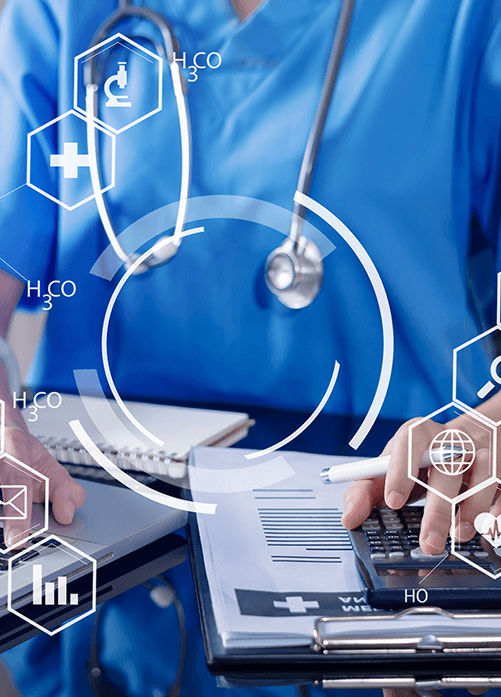
- Definition and Importance of Clinical Data Management
- Role of Data Management in Clinical Research
- Regulatory Guidelines and Compliance (e.g., ICH E6(R2))
- Phases of Clinical Trials
- Study Protocols and Case Report Forms (CRFs)
- Data Collection and Entry Procedures
- Elements of a Data Management Plan
- Study Data Standardization (CDISC)
- Data Validation and Quality Control Procedures
- Electronic Data Capture (EDC) Systems
- Data Entry Guidelines and Practices
- Query Management and Resolution
- Data Cleaning Procedures
- Identification and Handling of Data Discrepancies
- Data Quality Metrics and Reporting
- Database Development and Design Principles
- Data Dictionary and Coding
- Database Lock and Freeze Procedures
- Integration of External Data (e.g., Lab Data, Imaging)
- Data Reconciliation and Consistency Checks
- Data Import and Export Procedures
- GCP and Data Management
- Audit Preparation and Responses
- Inspections and Regulatory Authority Interactions
- Adverse Event Reporting and Processing
- Safety Data Integration with Clinical Data
- Signal Detection and Reporting
- Utilizing EHR Data in Clinical Trials
- Challenges and Considerations with Real-World Data
- Data Privacy and Security Concerns
- Data Analysis and Reporting
- Preparation for Regulatory Submissions (e.g., FDA, EMA)
- Interim and Final Clinical Study Reports (CSRs)
- Artificial Intelligence and Machine Learning in Data Management
- Blockchain and Data Security
- Future Directions in Clinical Data Management
- Quizzes and Knowledge Checks
- Practical Exercises (e.g., Data Cleaning, DMP Development)
- Group Projects and Presentations
- Final Exam or Capstone Project
- Mock Audits and Inspections
- Hands-On EDC System Training
- Guest Lectures from Industry Experts
This curriculum provides a comprehensive overview of Clinical Data Management, covering both theoretical knowledge and practical skills necessary for professionals in the field. Remember to adapt and update the curriculum to incorporate the latest technologies, regulatory guidelines, and industry best practices.
Career Paths
Clinical Data Management (CDM) is a critical component of clinical research, involving the collection, cleaning, and management of data from clinical trials. Here are some common career paths in Clinical Data Management:
- Responsibilities: Assist in data collection, validation, and cleaning activities. Ensure data quality and compliance with protocols.
- Skills Needed: Attention to detail, organizational skills, familiarity with clinical trial processes.
- Responsibilities: Oversee the data management process, develop data management plans, and coordinate data collection and validation activities.
- Skills Needed: Project management skills, knowledge of data management tools, attention to detail.
- Responsibilities: Analyze and report on clinical trial data, identify trends, and generate data summaries for reporting.
- Skills Needed: Data analysis skills, statistical knowledge, proficiency in data analysis software.
- Responsibilities: Implement and oversee data quality control processes, ensure compliance with regulatory requirements and industry standards.
- Skills Needed: Quality assurance knowledge, attention to detail, analytical skills.
- Responsibilities: Design and program electronic data capture (EDC) systems, generate data listings and reports.
- Skills Needed: Programming skills (e.g., SAS, SQL), database management knowledge, attention to detail.
- Responsibilities: Develop and maintain data standards, ensure consistency and compliance with industry standards (e.g., CDISC).
- Skills Needed: Knowledge of data standards, attention to detail, familiarity with CDISC standards.
- Responsibilities: Apply advanced data analysis techniques to generate insights from clinical trial data, contribute to study design and protocol development.
- Skills Needed: Statistical knowledge, data analysis skills, clinical research expertise.
- Responsibilities: Oversee the overall data management operations, lead a team of data managers, and ensure timely and high-quality data deliverables.
- Skills Needed: Leadership skills, project management expertise, strategic thinking.
- Responsibilities: Conduct audits to ensure compliance with data management processes, identify areas for improvement, and implement quality control measures.
- Skills Needed: Auditing skills, quality assurance knowledge, attention to detail.
- Responsibilities: Develop and implement data governance policies and procedures, ensure data integrity and compliance with regulatory requirements.
- Skills Needed: Governance knowledge, attention to detail, regulatory expertise.
- Responsibilities: Provide training on data management principles, tools, and processes to clinical research professionals.
- Skills Needed: Communication skills, knowledge of data management practices.
- Responsibilities: Offer specialized data management services to pharmaceutical companies, clinical research organizations, or research institutions.
- Skills Needed: Expertise in data management, business acumen, networking skills.
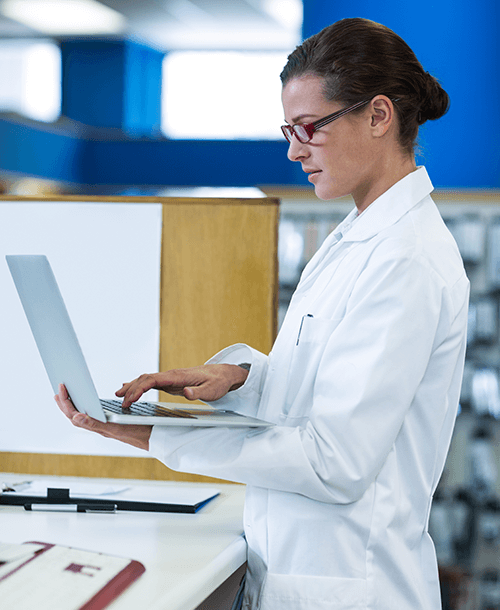
Remember that career paths in Clinical Data Management can evolve with experience and may lead to roles in project management, regulatory affairs, or other areas within the clinical research industry. Continuous professional development and staying updated with industry trends and technologies are important for success in this field.